The regional and industrial dimensions of gender inequality in firm top management positions: A classification tree and random forest analysis
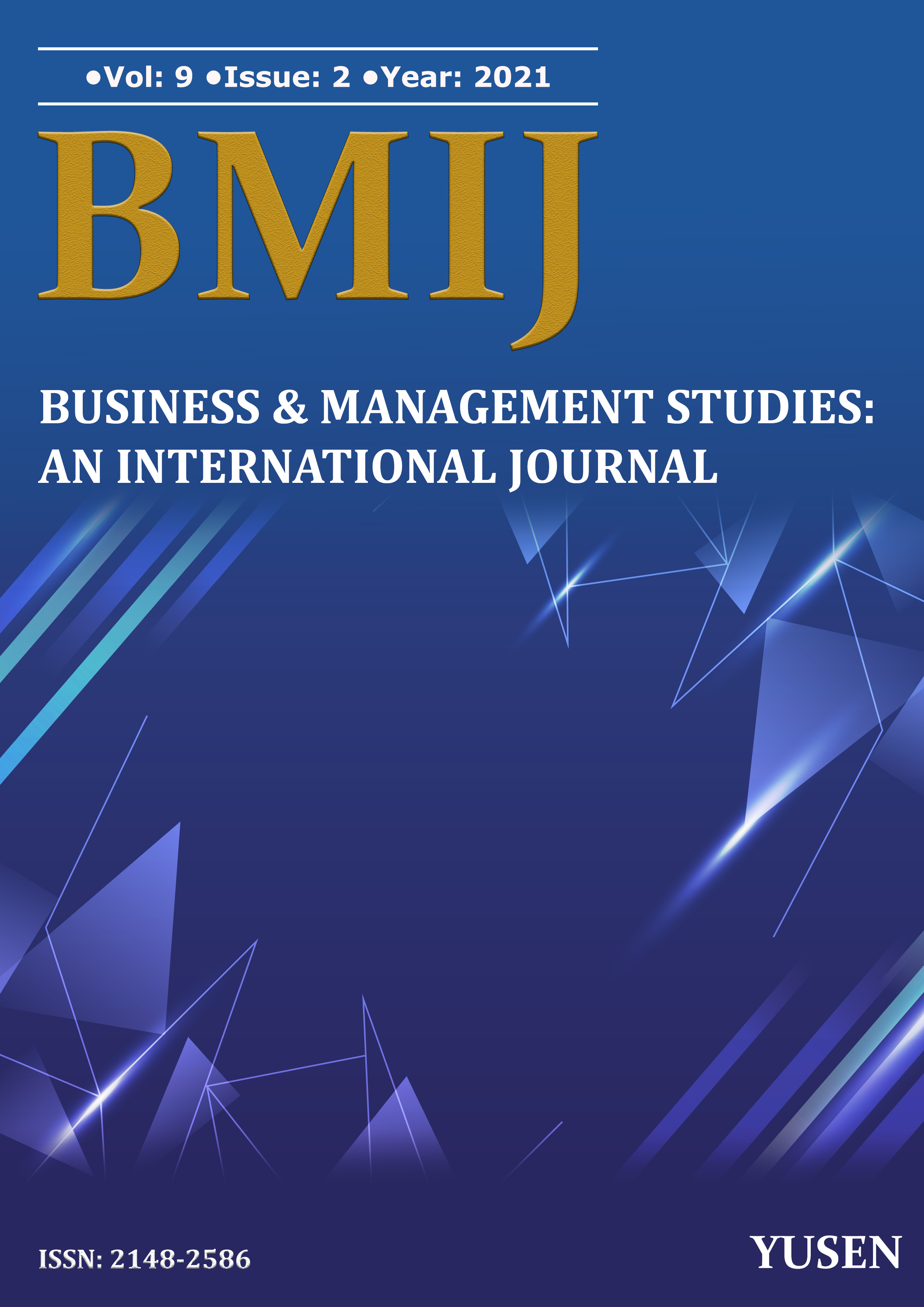
Published 2021-06-25
Keywords
- Ekonomik Coğrafya, Cinsiyet Eşitsizliği, Firmalar, Üst Yönetim, Makine Öğrenmesi.
- Economic Geography, Gender Inequality, Firms, Top Management, Machine Learning.
How to Cite
Copyright (c) 2021 Mehmet Güney Celbiş

This work is licensed under a Creative Commons Attribution-NonCommercial-NoDerivatives 4.0 International License.
Abstract
The issue of gender inequality in the labour market is a topic attracting attention in Turkey and globally. One specific manifestation of gender inequality is observed in relation to top management positions. In the present study, the underlying effects that create gender inequality in top management positions are examined to identify the conditions that lead to an unbalanced distribution. Using tree-based machine learning methods, the present study identifies the institutional, industrial, and regional attributes related to gender inequality in top management positions of firms in Turkey. Alongside numerous other findings, we observe that the top managers in firms facing problems related to crime, corruption, access to land and licenses, and establishments located in Northeast and Southeast Anatolia tend to be male. In addition, we also observe that under certain regional and institutional conditions, top female managers are more common in the retail, restaurant and hotel, textiles, clothing, and manufacturing industries. Together with identifying the regions and industries with the highest female-male inequality, the findings also highlight the institutional factors that lead to these inequalities. The results, alongside opening new research paths regarding the analysis of specific determinants, also have the potential of helping the development of new policies to tackle the problem. In the models presented in this study, data on 162 firms and 254 variables presented in the Enterprise Survey-2019 are analyzed using algorithmic models. In addition to the content-related relevance of the findings, the present study presents tree-based machine learning methods as new methodological alternatives concerning assessing the research question.
Downloads
References
- Adams, R. B., ve Funk, P. (2012). Beyond the glass ceiling: Does gender matter? Management Science, 58(2):219–235.
- Alpaydın, E. (2011). Yapay öğrenme. Boğaziçi Üniversitesi Yayınevi.
- Athey, S. (2018). The impact of machine learning on economics. In The economics of artificial intelligence: An agenda, 507–547. University of Chicago Press.
- Athey, S., ve Imbens, G. W. (2019). Machine learning methods that economists should know about. Annual Review of Economics, 11.
- Atkinson, E. J., ve Therneau, T. M. (2000). An introduction to recursive partitioning using the RPART routines. Rochester: Mayo Foundation.
- Ayyıldız Ünnü, N. A., Baybars, M. ve Kesken, J. (2014). Türkiye’de kadınların üniversiteler bağlamında yetki ve karar verme mekanizmalarına katılımı. Dumlupınar Üniversitesi Sosyal Bilimler Dergisi, (42):121–134.
- Bang, J., Sen, T., ve Basuchoudhary, A. (2017). New tools for predicting economic growth using machine learning: A guide for theory and policy.
- Barczak, G., Lassk, F., ve Mulki, J. (2010). Antecedents of team creativity: An examination of team emotional intelligence, team trust and collaborative culture. Creativity and Innovation Management, 19(4):332–345.
- Birleşmiş Milletler (2019), The Sustainable Development Goals Report 2019. New York.
- Becic, M. ve Vojinic, P. (2018). The role of female top manager in innovation activities: Case of Ceecs’ firms. Proceedings of the 10th Economics & Finance Conference, Rome kitabı içinde. International Institute of Social and Economic Sciences.
- Berber, M., ve Eser, B. Y. (2008). Türkiye’de kadın istihdam: ülke ve bölge düzeyinde sektörel analiz. ISGUC The Journal of Industrial Relations and Human Resources, 10(2):1–16.
- Black, D. A., Kolesnikova, N., ve Taylor, L. J. (2014). Why do so few women work in New York (and so many in Minneapolis)? Labor supply of married women across US cities. Journal of Urban Economics, 79:59–71.
- Boden, R. J. (1999). Gender inequality in wage earnings and female self-employment selection. The Journal of Socio-Economics, 28(3):351–364.
- Breiman, L. (1996). Bagging predictors. Machine Learning, 24(2):123–140.
- Breiman, L. (2001). Random forests. Machine Learning, 45(1):5–32.
- Breiman, L., Friedman, J. H., Olshen, R. A., ve Stone, C. J. (1984). Classification and Regression Trees. Wadsworth and Brooks, Monterey, CA.
- Brenner, S.-O., ve Levi, L. (1987). Long-term unemployment among women in Sweden. Social Science & Medicine, 25(2):153–161.
- Briel, S., ve Töpfer, M. (2020). The gender pay gap revisited: Does machine learning offer new insights? LASER.
- Campbell, K., ve Minguez-Vera, A. (2007). Gender diversity in the boardroom and firm financial performance. Journal of Business Ethics, 83(3):435–451.
- Can, Y., ve Eyidiker, U. (2019). Türkiye’de kadın istihdam profilinin istatistiksel bölgeler arasındaki farklıkların incelenmesi. XIII. IBANESS İktisat, İşletme ve Yönetim Bilimleri Kongreler Serisi – Tekirdağ-Güz / Türkiye. 05-06 Ekim 2019
- Chang, H.-J., Shih, Y.-S., ve Su, T.-J. (2014). Split selection methods for regression tree on detecting regional economic convergence. Journal of the Chinese Statistical Association, 52(2):180–208.
- Cohen, P. N. and Huffman, M. L. (2007). Working for the woman? Female managers and the gender wage gap. American Sociological Review, 72(5):681–704.
- Cook, A., ve Glass, C. (2013). Glass cliffs and organizational saviors. Social Problems, 60(2):168–187.
- Cotter, D. A., Hermsen, J. M., Ovadia, S., ve Vanneman, R. (2001). The glass ceiling effect. Social Forces, 80(2):655–681.
- Dayoglu, M., ve Kırdar, M. G. (2010). Türkiye’de kadınların işgücüne katılımında belirleyici etkenler ve eğilimler. DPT Yayınları, Ankara.
- Friedman, J., Hastie, T., ve Tibshirani, R. (2001). The Elements of Statistical Learning, volume 1. Springer Series in Statistics, New York.
- Friedman, J. H. (2001). Greedy function approximation: A gradient boosting machine. Annals of Statistics, 5:1189–1232.
- Géron, A. (2019). Hands-On Machine Learning with Scikit-Learn, Keras, and TensorFlow: Concepts, Tools, and Techniques to Build Intelligent Systems. O’Reilly Media.
- Glaeser, E. L., Kominers, S. D., Luca, M., ve Naik, N. (2018). Big data and big cities: The promises and limitations of improved measures of urban life. Economic Inquiry, 56(1):114–137.
- Goldstein, A., Kapelner, A., Bleich, J., ve Pitkin, E. (2015). Peeking inside the black box: Visualizing statistical learning with plots of individual conditional expectation. Journal of Computational and Graphical Statistics, 24(1):44–65.
- Greenwell, B. M. (2017). pdp: An R package for constructing partial dependence plots. The R Journal, 9(1):421–436.
- Harding, M. and Hersh, J. (2018). Big data in economics. IZA World of Labor, (451).
- Iman, H. S., ve Tarasyev, A. (2018). Machine learning methods in individual migration behavior. In Russian Regions in the Focus of Changes: Conference proceedings. Ekaterinburg, 2018, 72–81. LLC Publishing office EMC UPI.
- Islam, A., ve Amin, M. (2015). Women managers and the gender-based gap in access to education: Evidence from firm-level data in developing countries. Feminist Economics, 22(3):127–153.
- Jacobs, J. A. (1995). Gender Inequality at Work. ERIC.
- James, G., Witten, D., Hastie, T., ve Tibshirani, R. (2013). An Introduction to Statistical Learning, volume 112. Springer, New York.
- Kadın Girişimciler Derneği ve Türk Tuborg (2019). Türkiye Kadın Girişimcilik Endeksi-2019 Araştırma Raporu.
- Kaiser, M. (2018). Segmentation in urban labor markets: a machine learning application and a contracting perspective.
- Karatepe, S., ve Arıbaş, N. N. (2017). İş hayatında kadın yöneticilere ilişkin cinsiyet ayrımcılığı: Türkiye için bir değerlendirme. Yasama Dergisi, (31):7–23.
- Karcıoğlu, F. ve Leblebici, Y. (2014). Kadın Yöneticilerde Kariyer Engelleri: "Cam Tavan Sendromu" Üzerine Bir Uygulama. Atatürk University Journal of Economics & Administrative Sciences, 28(4).
- Kuhn, M. (2008). Building predictive models in R using the caret package. Journal of Statistical Software, 28(5):1–26.
- Liaw, A., ve Wiener, M. (2002). Classification and regression by randomforest. R News, 2(3):18–22.
- Liu, Y. (2019). Predicting labor market competition and employee mobility - a machine learning approach. PhD Dissertation, University of Iowa.
- Lopez-Yucra, K., Libaque-Saenz, C., Lazo, J., ve Bravo, E. (2018). Could machine learning improve the prediction of child labor in Peru? In Information Management and Big Data: 4th Annual International Symposium, SIMBig 2017, Lima, Peru, September 4-6, 2017, Revised Selected Papers, volume 795, 15. Springer.
- Mittal, M., Goyal, L. M., Sethi, J. K., ve Hemanth, D. J. (2019). Monitoring the impact of economic crisis on crime in India using machine learning. Computational Economics, 53(4):1467–1485.
- Mullainathan, S., ve Spiess, J. (2017). Machine learning: An applied econometric approach. Journal of Economic Perspectives, 31(2):87–106.
- Nyman, R., ve Ormerod, P. (2017). Predicting economic recessions using machine learning algorithms. arXiv preprint arXiv:1701.01428.
- Nyman, R., ve Ormerod, P. (2020). Understanding the great recession using machine learning algorithms. arXiv preprint arXiv:2001.02115.
- Örücü, E./ Kılıç R./ Kılıç T. (2007), “Cam tavan sendromu ve kadınların üst düzey yönetici pozisyonuna yükselmelerindeki engeller: Balıkesir ili örneği,” Celal Bayar Üniversitesi İ.İ.B.F. Yönetim ve Ekonomi Dergisi, 14 /2: 117-135
- Öztürk, S. (2018). Türkiye’de kadın girişimciliğinin iktisadi ve kültürel normlar çerçevesinde değerlendirilmesi. Girişimcilik ve Kalkınma Dergisi, 13(1):1–11.
- Ritter-Hayashi, D., Vermeulen, P. A. M., ve Knoben, J. (2016). Gender diversity and innovation: The Role of Women’s Economic Opportunity in Developing Countries. (DFID çalışma tebliği). Radboud University Nijmegen.
- Robinson, C., Hohman, F., ve Dilkina, B. (2017). A deep learning approach for population estimation from satellite imagery. In Proceedings of the 1st ACM SIGSPATIAL Workshop on Geospatial Humanities, 47–54.
- Rutherford, S. (2001). Organizational cultures, women managers and exclusion. Women in Management Review, 16(8):371–382.
- Şahin, F. (2011). Kadınların siyasal katılımlar çerçevesinde kadın meclislerinin yerel siyasetteki etkinlikleri ve üye profilleri. TC Başbakanlık Kadın Statüsü Genel Müdürlüğü, (Uzmanlık Tezi), Ankara.
- Shrader, C. B., Blackburn, V. B., ve Iles, P. (1997). Women in management and firm financial performance: An exploratory study. Journal of managerial issues, 355–372.
- Smith, N., Smith, V., ve Verner, M. (2006). Do women in top management affect firm performance? a panel study of 2, 500 Danish firms. International Journal of Productivity and Performance Management, 55(7):569–593.
- Sutton, C. D. (2005). Classification and regression trees, bagging, and boosting. In Handbook of Statistics, 303–329. Elsevier.
- Taşkın, E. (2012). Kadın yöneticilerin cam tavan algısının cam tavan aşma stratejilerine etkisi: Bursa örneği. Dumlupınar Üniversitesi Sosyal Bilimler Dergisi, 1(33):19–34.
- The World Bank (2019) Turkey - Enterprise Survey (ES) 2019, Ref. TUR_2019_ES_v01_M. https://www.enterprisesurveys.org/portal/login.aspx.
- Varian, H. R. (2014). Big data: New tricks for econometrics. Journal of Economic Perspectives, 28(2):3–28.
- Verma, C., Tarawneh, A. S., Illes, Z., Stoffova, V., ve Dahiya, S. (2018). Gender prediction of the European school’s teachers using machine learning: Preliminary results. In 2018 IEEE 8th International Advance Computing Conference (IACC). IEEE.
- Xu, W., Li, Z., Cheng, C., ve Zheng, T. (2013). Data mining for unemployment rate prediction using search engine query data. volume 7, 33–42. Springer.
- Yıldız, S., Alhas, F., Sakal, Ö., ve Yıldız, H. (2016). Cam Uçurum: Kadın Yöneticiler Cam Tavanı Ne Zaman Aşar? Ankara Üniversitesi SBF Dergisi, 71 (4), 1119-1146.
- Zhang, C., ve Zhang, J. (2014). Analysing Chinese citizens’ intentions of outbound travel: a machine learning approach. Current Issues in Tourism, 17(7):592–609.