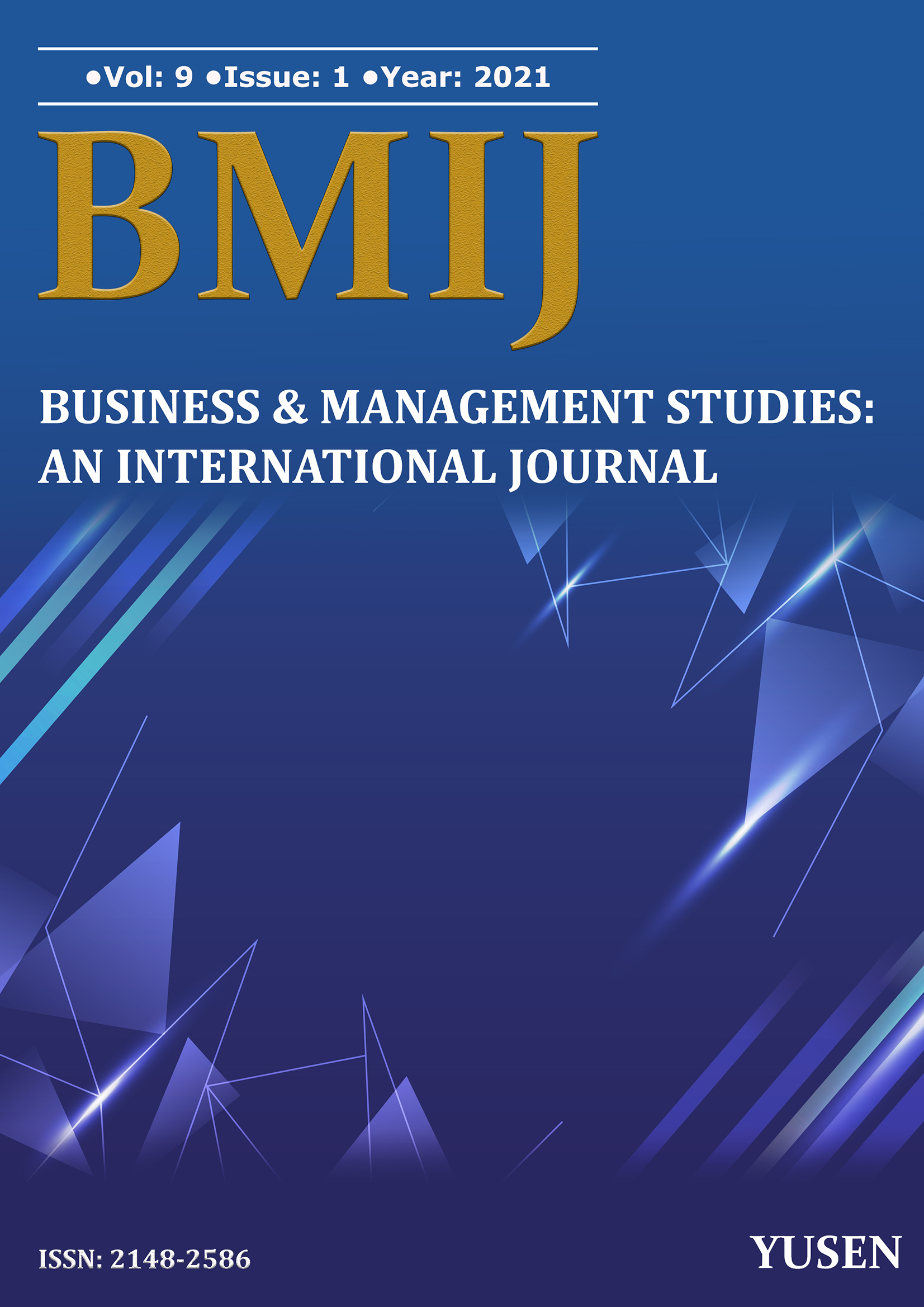
Published 2021-03-25
Keywords
- Deposit Banks, Cluster Analysis, Financial Ratios, Expectation-Maximization Algorithm Jel Codes: C38, G0
- Mevduat Bankaları, Kümeleme Analizi, Finansal Oranlar, Beklenti Maksimizasyonu Algoritması
How to Cite
Copyright (c) 2021 Meltem KARAATLI- Ece YILDIZ

This work is licensed under a Creative Commons Attribution-NonCommercial-NoDerivatives 4.0 International License.
Abstract
Banks have an essential role among financial institutions; their capital must be at a certain level. It is impossible for banks that do not have sufficient capital to carry out their main activities, such as deposit collection and borrowing. Finding substantial capital is the cornerstone of a robust financial structure. For this purpose, in this study, the banks' financial structures were analysed by clustering analysis with the financial ratios obtained using the data of 20 deposit banks operating in the banking sector as of 2017. The expectation-maximisation Algorithm was used for cluster analysis. As a result of the study, financially similar banks are presented and interpreted. After the clustering analysis, a significant difference between clusters in terms of each variable was examined by One Way ANOVA and Kruskal Wallis tests. When the clustering results are examined, it is seen that the ownership structures of banks (public, private, foreign) do not have a full effect on cluster formation
Downloads
References
- Akpınar, H. (2000). Veri tabanlarında Bilgi Keşfi ve Veri Madenciliği. I.U. Işletme Fakültesi Dergisi, 29(1), 1-22.
- Ali, F., M., N., & Hamed, A., A., M. (2018). Usage Apriori And Clustering Algorithms In WEKA Tools To Mining Dataset Of Traffic Accidents. Journal Of Information and Telecommunication, 2(3), 231-245.
- Amel, D., & Rhoades, S. (1988). Strategic Groups in Banking. The Review of Economics and Statistics, 70(4), 685-689.
- Ceylan, Z., Gürsev S., Bulkan S. (2017). İki Aşamalı Kümeleme Analizi ile Bireysel Emeklilik Sektöründe Müşteri Profilinin Değerlendirilmesi. Bilişim Teknolojileri Dergisi, 10(4), 475-485.
- Chawla, D., Joshi H., (2016). Consumer perspectives about mobile banking adoption in India – a cluster analysis, IJBM 35,4, 616.
- Dağ, O., Karaatlı, M., (2020). Resort Otellerin Kümeleme Analizi İle İncelenmesi: Antalya İli Örneği. Süleyman Demirel Üniversitesi Sosyal Bilimler Enstitüsü Dergisi, 1(36), 200-232
- Dias J., Ramos S., (2014). The aftermath of the subprime crisis: a clustering analysis of world banking sector. Rev Quant Finan Acc, 42, 293–308.
- Doğan, M., (2013). Katılım ve Geleneksel Bankaların Finansal Performanslarının Karşılaştırılması: Türkiye Örneği. Muhasebe Finansman Dergisi, 175-187.
- Ferstl R., Seres D., (2012). Clustering Austrian Bahwetanks' Business Models and Peer Groups in the European Banking Sector. Fınancal Stabılıty Report 24 – December, 79-95.
- Jagric T., Bojnec S., Jagric V. (2015). Optimised spiral spherical self-organising map approach to sector analysis – The case of banking. Expert Systems with Applications 42, 5531-5540.
- Kalikov, A. (2006). Veri Madenciliği ve Bir E-Ticaret Uygulaması, Yüksek Lisans Tezi, Gazi Üniversitesi, Fen Bilimleri Enstitüsü, Ankara.
- Karaatlı, M., Karataş T., Ömürbek, N., (2020). Ülkelerin İnsani Özgürlük Endeksine Göre Kümelenmesi. Anadolu Üniversitesi Sosyal Bilimler Enstitüsü Dergisi, 271-286
- Karaatlı, M., Altıntaş, E., (2018). Borsa İstanbul İşletmelerinin Veri Madenciliği İle Kümelenmesi. Mehmet Akif Ersoy Üniversitesi Sosyal Bilimler Enstitüsü Dergisi, 10(26), 871-886
- Köçek, G., Cinser, V. (2008). Türkiye’de faaliyette bulunan Ticaret Bankalarının Performanslarına Göre sınıflandırılmasında Etkili olan Değişkenlerin Belirlenmesi ve Bir Uygulama Denemesi. Dumlupınar Üniversitesi Sosyal Bilimler Dergisi, 22, 189- 206.
- Lueg, R., Schmaltz, C., & Tomkus, M.(2019). Business Models In Banking: A Cluster Analysis Using Archival Data, Trames, 23(73/68), 1, 79-107.
- Molinera, M., Apellainz, P., & Cinca, S. (1993). A Multivariate Analysis of Spanish Bond Ratings. International Journal of Management Sciences, 24(4), 451-462.
- Patterson, D., Liu, F., Turner, D., Concepcion, A., & Lynch, R. (2008, March). Performance Comparison of the Data Reduction System. Proceedings of the SPIE Symposium on Defense and Security, Orlando, FL.,27-34.
- Prajwala, T., R.,& Sangeeta, V., I. (2014). Comparative Analysis of EM Clustering Algorithm and Density Based Clustering Algorithm Using WEKA tool. International Journal of Engineering Research and Development, 9(8), 19-24.
- Sharma, N., Bajpai, A., & Litoriya, M., R .(2012). Comparison the Various Clustering Algorithms of Weka Tools. International Journal of Emerging Technology and Advanced Engineering, 2(5), 73-80.
- TBB. Bankalarımız 2017 Kitabı. (2017). http://www.tbb.org.tr/_statistiki Raporlar (Erişim Tarihi, 15 Haziran 2020).
- Vagizova, V., Lurie K., Ivasiv, I., (2014). Clustering of Russian banks: business models of interaction of the banking sector and the real economy. Problems and Perspectives in Management, 12(1), 83-93.
- Widya, P.A., & Sudarma, M. (2018). Implementation of EM Algorithm in Data Mining for Clustering Female Cooperative. International Journal of Engineering and Emerging Technology, 3(1), 75-79.
- Witten I.H., Frank E., & Hall Ma. (2011). Data mining: practical machine learning tools and techniques. Elsevier, London.
- https://www.tmsf.org.tr/tr/Tmsf/Mevduat/mevduat.sss, Erişim 25.12.2020
- https://www.tbb.org.tr/tr/bankacilik/arastirmaveyayinlar/kitaplar/kitaplar/55?year=2017 Erişim: 25.12.2020