Selection of robot in industry 4.0: A case study on metal kitchenware manufacturing with the integration of SWARA and fuzzy TOPSIS methods
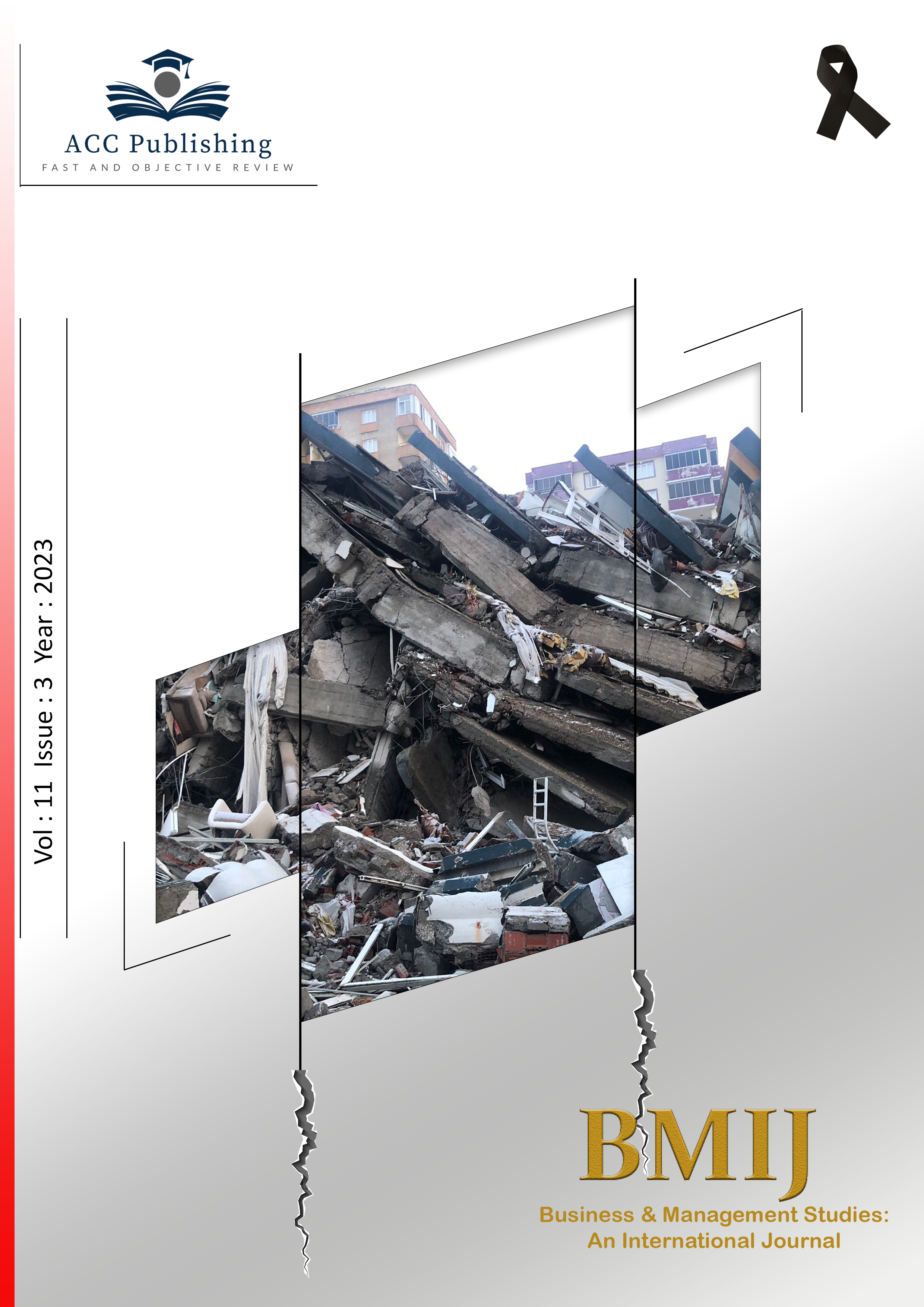
Published 2023-09-24
Keywords
- Endüstri 4.0, Akıllı Robot, SWARA, Bulanık TOPSIS
- Industry 4.0, Smart Robot, SWARA, Fuzzy TOPSIS
How to Cite
Copyright (c) 2023 Mehri Banu Erdem- Alaeddin Koska

This work is licensed under a Creative Commons Attribution-NonCommercial-NoDerivatives 4.0 International License.
How to Cite
Abstract
Industrial manufacturing constantly evolves to help manufacturers meet growing customer demand and remain competitive in the global marketplace. Robotic applications in manufacturing produce more protection, quality and sustainability for businesses. Many manufacturing processes in the industry have been facilitated by smart robots working with great precision and speed. This study aimed to solve a manufacturing company's robot evaluation and selection problem. Another purpose is to determine the importance of robot selection criteria for this business. The company is a large-scale company that manufactures metal kitchenware in Kahramanmaraş. This company wants to replace its machine with a smart robot and invest in a more functional one. However, since the investment in smart robots is a high-cost and financially and morally expensive investment, it is difficult to decide between the options. At this point, the study's main purpose is to support the business in the selection decision. In the study, SWARA and fuzzy TOPSIS methods, which are multi-criteria decision-making methods, were integrated and used. There are three alternative smart robots that the firm wants to choose from. As a result of the analysis, one of these alternatives was suggested as the best choice for the firm.
References
- Agarwal, S., Chakraborty, S., Prasad, K., & Chakraborty, S. (2021). A rough multi-attributive border approximation area comparison approach for arc welding robot selection. Jordan Journal of Mechanical & Industrial Engineering, 15(2). 169-180.
- Aliakbari Nouri, F., Khalili Esbouei, S., ve Antucheviciene, J. (2015). A hybrid MCDM approach based on fuzzy ANP and fuzzy TOPSIS for technology selection. Informatica, 26(3), 369-388.
- Alinezhad, A., Makui, A., Kiani Mavi, R., & Zohrehbandian, M. (2011). An MCDM-DEA approach for technology selection. Journal of Industrial Engineering International, 7(12), 32-38. 10.30495/JIEI.2022.1944196.1175
- Athawale, V. M., & Chakraborty, S. (2011). A comparative study on the ranking performance of some multi-criteria decision-making methods for industrial robot selection. International journal of industrial engineering computations, 2(4), 831-850. doi: 10.5267/j.ijiec.2011.05.002
- Bader, F., & Rahimifard, S. (2020). A methodology for the selection of industrial robots in food handling. Innovative Food Science & Emerging Technologies, 64, 102379. https://doi.org/10.1016/j.ifset.2020.102379
- Bao, H., & Shi, X. (2022). Robot selection using an integrated MAGDM model based on ELECTRE method and linguistic q-rung orthopair fuzzy information. Mathematical Problems in Engineering, 2022. https://doi.org/10.1155/2022/1444486
- Bhangale, P. P., Agrawal, V. P., & Saha, S. K. (2004). Attribute based specification, comparison and selection of a robot. Mechanism and Machine Theory, 39(12), 1345-1366. https://doi.org/10.1016/j.mechmachtheory.2004.05.020
- Bhattacharya, A., Sarkar, B., ve Mukherjee, S. K. (2005). Integrating AHP with QFD for robot selection under requirement perspective. International journal of production research, 43(17), 3671-3685. DOI: 10.1080/00207540500137217
- Bairagi, B., Dey, B., Sarkar, B., & Sanyal, S. (2014). Selection of robot for automated foundry operations using fuzzy multi-criteria decision making approaches. International Journal of Management Science and Engineering Management, 9(3), 221-232. https://doi.org/10.1080/17509653.2014.880076
- Braglia, M., & Petroni, A. (1999). Evaluating and selecting investments in industrial robots. International Journal of Production Research, 37(18), 4157-4178. https://doi.org/10.1080/002075499189718
- Chatterjee, P., Athawale, V. M., & Chakraborty, S. (2010). Selection of industrial robots using compromise ranking and outranking methods. Robotics and Computer-Integrated Manufacturing, 26(5), 483-489. https://doi.org/10.1016/j.rcim.2010.03.007
- Chen, C. T. (2000). Extensions of the TOPSIS for group decision-making under fuzzy environment. Fuzzy sets and systems, 114(1), 1-9. https://doi.org/10.1016/S0165-0114(97)00377-1
- Chodha, V., Dubey, R., Kumar, R., Singh, S., & Kaur, S. (2022). Selection of industrial arc welding robot with TOPSIS and Entropy MCDM techniques. Materials Today: Proceedings, 50, 709-715. https://doi.org/10.1016/j.matpr.2021.04.487
- Chu, T. C., & Lin, Y. C. (2003). A fuzzy TOPSIS method for robot selection. The International Journal of Advanced Manufacturing Technology, 21, 284-290. https://doi.org/10.1007/s001700300033
- Costa, D. S., Mamede, H. S., & da Silva, M. M. (2023). A method for selecting processes for automation with AHP and TOPSIS. Heliyon, 9(3).
- Devi, K. (2011). Extension of VIKOR method in intuitionistic fuzzy environment for robot selection. Expert Systems with Applications, 38(11), 14163-14168. https://doi.org/10.1016/j.eswa.2011.04.22
- Evans, L., Lohse, N., ve Summers, M. (2013). A fuzzy-decision-tree approach for manufacturing technology selection exploiting experience-based information. Expert systems with applications, 40(16), 6412-6426. https://doi.org/10.1016/j.eswa.2013.05.047
- Ghorabaee, M. K. (2016). Developing an MCDM method for robot selection with interval type-2 fuzzy sets. Robotics and Computer-Integrated Manufacturing, 37, 221-232. https://doi.org/10.1016/j.rcim.2015.04.007
- Gitinavard, H., Mousavi, S. M., Vahdani, B., & Siadat, A. (2016). A new distance-based decision model in interval-valued hesitant fuzzy setting for industrial selection problems. Scientia Iranica, 23(4), 1928-1940. 10.24200/SCI.2016.3938
- Goel, R., ve Gupta, P. (2020). Robotics and industry 4.0. a roadmap to ındustry 4.0: smart production, Sharp Business and Sustainable Development, 157-169. https://doi.org/10.1007/978-3-030-14544-6_9
- Hamzeh, R., & Xu, X. (2019). Technology selection methods and applications in manufacturing: A review from 1990 to 2017. Computers & Industrial Engineering, 138, 106123. https://doi.org/10.1016/j.cie.2019.106123
- Huang, Z., Shen, Y., Li, J., Fey, M., ve Brecher, C. (2021). A survey on AI-driven digital twins in industry 4.0: Smart manufacturing and advanced robotics. Sensors, 21(19), 6340. https://doi.org/10.3390/s21196340
- Hwang, C. L., Yoon, K., Hwang, C. L., & Yoon, K. (1981). Methods for multiple attribute decision making. Multiple attribute decision making: methods and applications a state-of-the-art survey, 58-191. https://doi.org/10.1007/978-3-642-48318-9_3
- İç, Y. T., Yurdakul, M., Günyar, A., & Önel, H. (2017). Endüstriyel Robot Seçimi İçin Bir Karar Destek Sistemi. Makina Tasarım ve İmalat Dergisi, 15(2), 92-105.
- Iç, Y., Atalay, K., & Yurdakul, M. (2021). Kararsız Bulanık TOPSIS Yöntemi ile Endüstriyel Robot Seçimi. Bulanık Çok Kriterli Karar Verme Yöntemleri, Mehmet Kabak, Babek Erdebli (Ed.), Bulanık çok kriterli karar verme yöntemleri, Erişim adresi: https://avesis.gazi.edu.tr/yayin/16cb160c-d7c6-4d1e-8198-0e30077d003c/bulanik-cok-kriterli-karar-verme-yontemleri, Nobel, Ankara, 129-140.
- Jahanshahloo, G. R., Lotfi, F. H., & Izadikhah, M. (2006). Extension of the TOPSIS method for decision-making problems with fuzzy data. Applied mathematics and computation, 181(2), 1544-1551. https://doi.org/10.1016/j.amc.2006.02.057
- Javaid, M., Haleem, A., Singh, R. P., & Suman, R. (2021). Substantial capabilities of robotics in enhancing industry 4.0 implementation. Cognitive Robotics, 1, 58-75. https://doi.org/10.1016/j.cogr.2021.06.001
- Kao, C., & Liu, S. T. (2022). Group decision making in data envelopment analysis: A robot selection application. European Journal of Operational Research, 297(2), 592-599.
- Kahraman, C., Çevik, S., Ates, N. Y., ve Gülbay, M. (2007). Fuzzy multi-criteria evaluation of industrial robotic systems. Computers & Industrial Engineering, 52(4), 414-433. https://doi.org/10.1016/j.cie.2007.01.005
- Karande, P., Zavadskas, E., & Chakraborty, S. (2016). A study on the ranking performance of some MCDM methods for industrial robot selection problems. International Journal of Industrial Engineering Computations, 7(3), 399-422. DOI: 10.5267/j.ijiec.2016.1.001
- Karsak, E. E. (2008). Robot selection using an integrated approach based on quality function deployment and fuzzy regression. International Journal of Production Research, 46(3), 723-738. https://doi.org/10.1080/00207540600919571
- Keršuliene, V., Zavadskas, E. K., ve Turskis, Z. (2010). Selection of rational dispute resolution method by applying new step‐wise weight assessment ratio analysis (SWARA). Journal of business economics and management, 11(2), 243-258. Doi:10.3846/jbem.2010.12
- Kumar, V., Kalita, K., Chatterjee, P., Zavadskas, E. K., & Chakraborty, S. (2022). A SWARA-CoCoSo-based approach for spray painting robot selection. Informatica, 33(1), 35-54. 10.15388/21-INFOR466
- Mondal, S., & Chakraborty, S. (2013). A solution to robot selection problems using data envelopment analysis. International journal of industrial engineering computations, 4(3), 355-372. doi: 10.5267/j.ijiec.2013.03.007
- Mondal, S., Kuila, S., Singh, A. K., & Chatterjee, P. (2017). A complex proportional assessment method-based framework for industrial robot selection problem. Int J Res Sci Eng, 3(2), 368-378.
- Narayanamoorthy, S., Geetha, S., Rakkiyappan, R., & Joo, Y. H. (2019). Interval-valued intuitionistic hesitant fuzzy entropy based VIKOR method for industrial robots selection. Expert Systems with Applications, 2019, 121: 28-37. https://doi.org/10.1016/j.eswa.2018.12.015
- Nasrollahi, M., Ramezani, J., & Sadraei, M. (2020). A FBWM-PROMETHEE approach for industrial robot selection. Heliyon, 6(5), e03859. https://doi.org/10.1016/j.heliyon.2020.e03859
- Parameshwaran, R., Kumar, S. P., & Saravanakumar, K. (2015). An integrated fuzzy MCDM based approach for robot selection considering objective and subjective criteria. Applied Soft Computing, 26, 31-41. https://doi.org/10.1016/j.asoc.2014.09.025
- Piippo, P., Torkkeli, M., & Tuominen, M. (1999, July). Use of GDSS for selection of technology: new integrated CAD-system for an entire company. In PICMET'99: Portland International Conference on Management of Engineering and Technology. Proceedings Vol-1: Book of Summaries (IEEE Cat. No. 99CH36310) 1(13), IEEE. 10.1109/PICMET.1999.807689
- Rashid, T., Beg, I., & Husnine, S. M. (2014). Robot selection by using generalized interval-valued fuzzy numbers with TOPSIS. Applied Soft Computing, 21, 462-468. https://doi.org/10.1016/j.asoc.2014.04.002
- Rao, R. V. (2007). Decision making in the manufacturing environment: using graph theory and fuzzy multiple attribute decision making methods (Vol. 2, p. 294). London: Springer.
- Sampathkumar, S., Augustin, F., Kaabar, M. K., & Yue, X. G. (2023). An integrated intuitionistic dense fuzzy Entropy-COPRAS-WASPAS approach for manufacturing robot selection. Advances in Mechanical Engineering, 15(3), 16878132231160265.
- Sen, D. K., Datta, S., & Mahapatra, S. S. (2016). Extension of PROMETHEE for robot selection decision making: Simultaneous exploration of objective data and subjective (fuzzy) data. Benchmarking: An International Journal. 4(2013), 355-372. https://doi.org/10.1108/BIJ-08-2015-0081
- Shahrabi, M. (2014). identification and selection of robot using FAHP and FTOPSIS hybrid model. International Journal of Modern Engineering Sciences, 3(1), 16-28.
- Shih, H. S. (2008). Incremental analysis for MCDM with an application to group TOPSIS. European journal of operational research, 186(2), 720-734. https://doi.org/10.1016/j.ejor.2007.02.012
- Şahin, M. (2020). A Hybrid Multi-criteria Decision Approach for Industrial Robot Selection. Avrupa Bilim ve Teknoloji Dergisi, 1-9. https://doi.org/10.31590/ejosat.818275
- Wang, Y. J., & Lee, H. S. (2007). Generalizing TOPSIS for fuzzy multiple-criteria group decision-making. Computers & Mathematics with Applications, 53(11), 1762-1772. https://doi.org/10.1016/j.camwa.2006.08.037
- Wu, H. Y., Tzeng, G. H., & Chen, Y. H. (2009). A fuzzy MCDM approach for evaluating banking performance based on Balanced Scorecard. Expert systems with applications, 36(6), 10135-10147. https://doi.org/10.1016/j.eswa.2009.01.005
- Zhou, F., Wang, X., & Goh, M. (2018). Fuzzy extended VIKOR-based mobile robot selection model for hospital pharmacy. International Journal of Advanced Robotic Systems, 15(4), https://doi.org/10.1177/1729881418787315