Sigara içme davranışını tahmin etmede hibrit bir makine öğrenimi yaklaşımı: Türkiye örneği
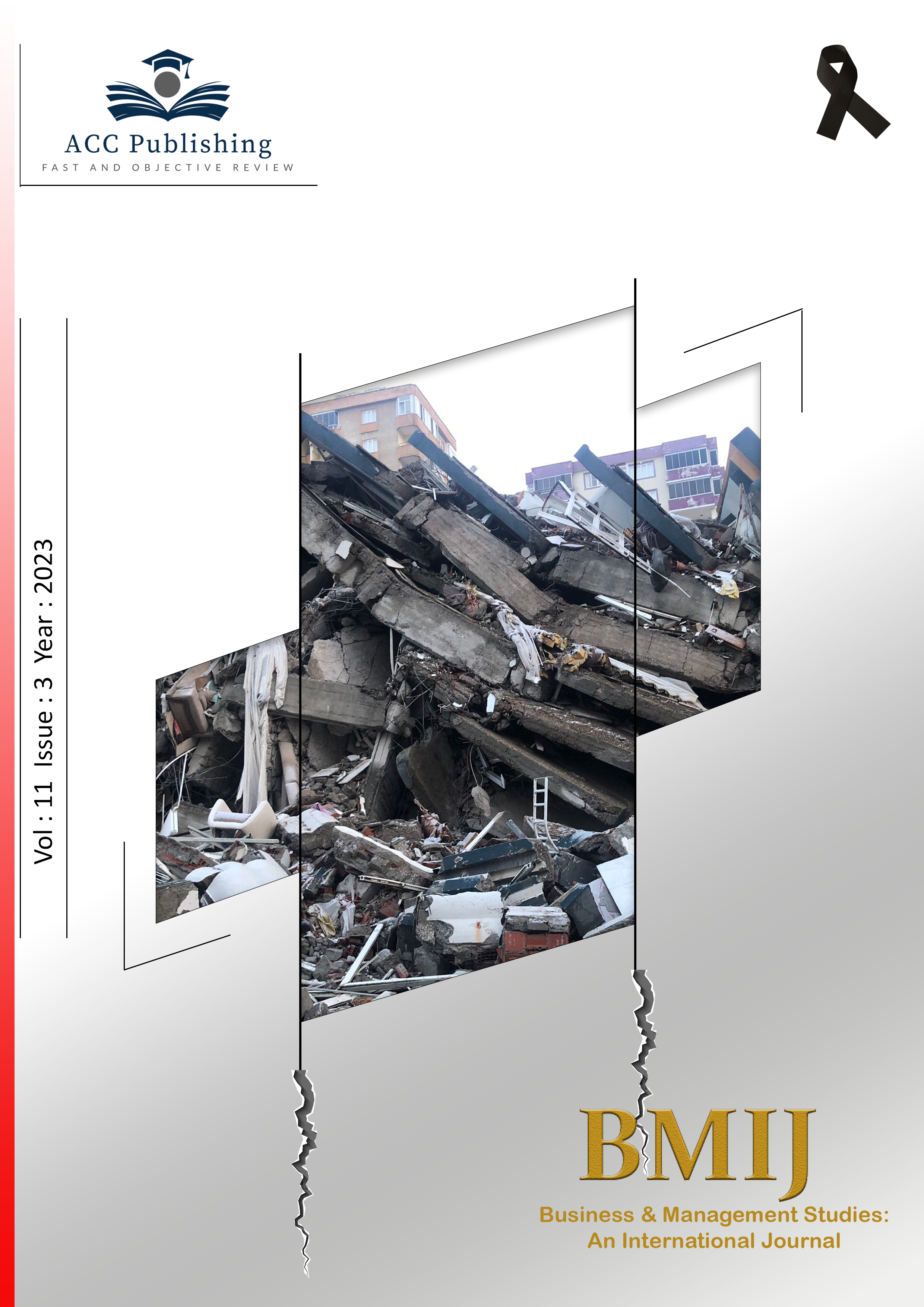
Yayınlanmış 24.09.2023
Anahtar Kelimeler
- Health Behaviour, Cross-Sectional Models, General
- Davranışsal Sağlık, Yatay Kesit Modelleri, Genel
Nasıl Atıf Yapılır
Telif Hakkı (c) 2023 Nuray Tezcan- Gökçe Karahan Adalı- Anıl Burcu Özyurt Serim

Bu çalışma Creative Commons Attribution-NonCommercial-NoDerivatives 4.0 International License ile lisanslanmıştır.
Nasıl Atıf Yapılır
Öz
Bu çalışma, Türkiye'de 15 yaş ve üstü kişilerin sigara içme davranışlarını hem denetimli hem de denetimsiz makine öğrenimi yöntemleri kullanarak analiz etmeyi amaçlamaktadır. Bu çalışmada, sigara içme davranışını tahmin etmek için C4.5 ve Random Forest (RF) eğitilmiş ve ayrıca ilişkileri tespit etmek için apriori algoritması kullanılmıştır. Denetlenen modellerin performanslarını karşılaştırmak için duyarlılık, özgüllük, doğruluk, pozitif tahmin değeri (ppv), f-ölçüsü kullanıldı. Sigara içme davranışını tahmin etmek ve sigarayı etkileyen faktörleri belirlemek için 17084 örneklem büyüklüğü ile Türkiye Sağlık Araştırması 2019 kullanılmıştır. Veri analizi ve performans değerlendirmesi RStudio tarafından R programlama dili ile yapılmıştır. Birliktelik kurallarına göre cinsiyet, yaş ve alkol tüketimi, sigara içme davranışının en temsili özellikleri olarak belirlenir. Dernekler sigara içme, içmeme ve sigarayı bırakma davranışında belirlendi. Ayrıca RF algoritmasının C4.5 algoritmasına göre daha iyi sonuçlara sahip olduğu görülmüştür. Sigara içme davranışını tahmin etmede 0,909 doğruluk, 0,965 özgüllük, 0,782 duyarlılık, 0,908 ppv, 0,840 f-ölçüsü ile daha iyi performans gösteren RF modelinin kullanılması tercih edilmiştir. Bu çalışma hem Türkiye'deki en kapsamlı ulusal sağlık araştırması verilerini kapsaması hem de bu veriler üzerinde makine öğrenmesi yöntemlerinin kullanılması açısından literatüre katkı sağlamakta ve bu tür veri setlerinin makine öğrenimi yöntemleriyle analiz edilebileceğini göstermektedir.
Referanslar
- Abo-Tabik, M., Benn, Y., & Costen, N. (2021). Are Machine Learning Methods the Future for Smoking Cessation Apps? Sensors, 21(13), 4254.
- Abo-Tabik, M., Costen, N., Darby, J., & Benn, Y. (2019, August). Decision Tree Model of Smoking Behaviour. In 2019 IEEE SmartWorld, Ubiquitous Intelligence & Computing, Advanced & Trusted Computing, Scalable Computing & Communications, Cloud & Big Data Computing, Internet of People and Smart City Innovation (SmartWorld/SCALCOM/UIC/ATC/CBDCom/IOP/SCI) (pp. 1746-1753). IEEE.
- Coughlin, L. N., Tegge, A. N., Sheffer, C. E., & Bickel, W. K. (2020). A machine-learning approach to predicting smoking cessation treatment outcomes. Nicotine and Tobacco Research, 22(3), 415-422.
- Ding, X., Yang, Y., Stein, E. A., & Ross, T. J. (2017). Combining multiple resting-state fMRI features during classification: optimized frameworks and their application to nicotine addiction. Frontiers in human neuroscience, 11, 362.
- Dumortier, A., Beckjord, E., Shiffman, S., & Sejdić, E. (2016). Classifying smoking urges via machine learning. Computer methods and programs in biomedicine, 137, 203-213.
- Durmuşoğlu, Z. & Kocabey Çiftçi, P. (2021). Socio-demographic determinants of smoking: A data mining analysis of the Global Adult Tobacco Surveys. Turkish Journal of Public Health, 19 (3), 251-262. DOI: 10.20518/tjph.884692
- Evenhuis, A., Occhipinti, S., Jones, L., & Wishart, D. (2023). Factors associated with cessation of smoking in health professionals: a scoping review. Global Health Action, 16(1). https://doi.org/10.1080/16549716.2023.2216068
- Genuer, R & Poggi, M. (2020) Random Forest with R. Use R! Springer.
- Goodchild, M., Nargis, N., & d'Espaignet, E. T. (2018). Global economic cost of smoking-attributable diseases. Tobacco control, 27(1), 58-64.
- Han, J. ve Kamber, M. (2006), Data mining: concepts and techniques (the Morgan Kaufmann Series in data management systems), 2nd Edition., Morgan Kaufmann Publishers, ISBN: 978-1-55860-901-3.
- Issabakhsh M, Sánchez-Romero LM, Le TTT, Liber AC, Tan J. (2023) Machine learning application for predicting smoking cessation among US adults: An analysis of waves 1-3 of the PATH study. PLOS ONE 18(6): e0286883. https://doi.org/10.1371/journal.pone.0286883
- Jitenkumar Singh, K., Jiran Meitei, A., Alee, N. T., Kriina, M., & Haobijam, N. S. (2022). Machine learning algorithms for predicting smokeless tobacco status among women in Northeastern States, India. International Journal of System Assurance Engineering and Management, 13(5), 2629-2639.
- Koslovsky, M. D., Swartz, M. D., Chan, W., Leon‐Novelo, L., Wilkinson, A. V., Kendzor, D. E., & Businelle, M. S. (2018). Bayesian variable selection for multistate Markov models with interval‐censored data in an ecological momentary assessment study of smoking cessation. Biometrics, 74(2), 636-644.
- Maginnity, J. D. (2020). Comparing the Uses and Classification Accuracy of Logistic and RF Models on an Adolescent Tobacco Use Dataset (Doctoral dissertation, The Ohio State University).
- Mak, K. K., Lee, K., & Park, C. (2019). Applications of machine learning in addiction studies: A systematic review. Psychiatry research, 275, 53-60.
- McCormick PJ, Elhadad N, Stetson PD. (2008) Use of semantic features to classify patient smoking status. AMIA Annu Symp Proc.; 450-454.
- Nollen NL, Ahluwalia JS, Lei Y, Yu Q, Scheuermann TS, Mayo MS. (2016) Adult Cigarette Smokers at Highest Risk for Concurrent Alternative Tobacco Product Use Among a Racially/Ethnically and Socioeconomically Diverse Sample. Nicotine Tob Res Off J Soc Res Nicotine Tob.;18(4):386-394
- RStudio, 2023, Home - RStudio, http://www.rstudio.com/, [Accessed: May 2023].
- Shearer, C. (2000). The CRISP-DM model: The new blueprint for data mining. Journal of Data Warehousing, 5(4), 13–22.
- Singh J., Meitei K., J., A., Alee, N. T., Kriina, M., & Haobijam, N. S. (2022). Machine learning algorithms for predicting smokeless tobacco status among women in Northeastern States, India. International Journal of System Assurance Engineering and Management, 13(5), 2629-2639.
- Singh, A., & Katyan, H. (2019). Classification of nicotine-dependent users in India: a decision-tree approach. Journal of Public Health, 27, 453-459.
- Thakur, S. S., Poddar, P., & Roy, R. B. (2022). Real-time prediction of smoking activity using machine learning based multi-class classification model. Multimedia Tools and Applications, 81(10), 14529-14551.
- TurkStat (2019), Turkey Health Interview Survey 2019
- United Nations (2015). Transforming our World: The 2030 Agenda for Sustainable Development. https://sustainabledevelopment.un.org/post2015/transformingourworld/publication
- WHO (2021). WHO report on the global tobacco epidemic 2021: addressing new and emerging products. https://www.who.int/publications/i/item/9789240032095.
- WHO (2022). Noncommunicable diseases. https://www.who.int/news-room/fact-sheets/detail/noncommunicable-diseases
- WHO (2023) “Obesity and overweight”, https://www.who.int/news-room/fact-sheets/detail/obesity-and-overweight [Accessed: January 2023]
- WHO. (2019). WHO global report on trends in prevalence of tobacco use 2000-2025. https://www.who.int/publications/i/item/who-globalreport-on-trends-in-prevalence-of-tobacco-use-2000-2025-third-edition
- Zhang, Y., Liu, J., Zhang, Z., & Huang, J. (2019). Prediction of daily smoking behaviour based on decision tree machine learning algorithm. In 2019 IEEE 9th International Conference on Electronics Information and Emergency Communication (ICEIEC) (pp. 330-333).