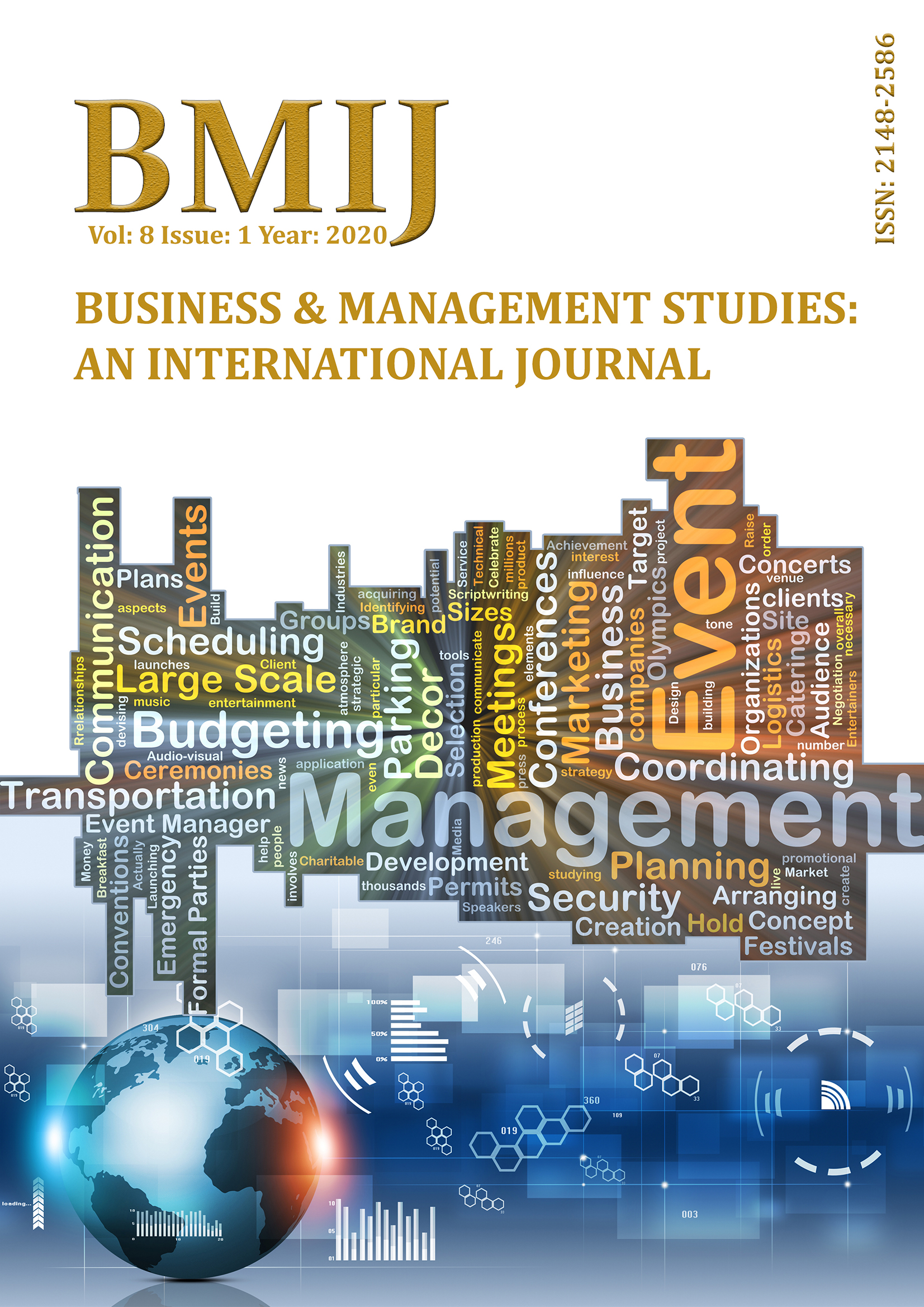
Yayınlanmış 25.03.2020
Anahtar Kelimeler
- Cryptocurrency, Lazy Learning, K-Star Algorithm, Classification
- Kripto Para, Lazy Learning, K-Star Algoritması, Sınıflandırma
Nasıl Atıf Yapılır
Nasıl Atıf Yapılır
Öz
Bitcoin en popüler ve yaygın olarak kullanılan dijital para birimidir. Bu nedenle, Bitcoin fiyat hareketinin tahmini finansal piyasalar için büyük önem taşımaktadır. Bitcoin fiyat tahmininde ekonometrik modellerin yanında veri madenciliği yöntemlerinden de faydalanılmaktadır. Veri madenciliğinde kullanılan araç ve yöntemler yardımıyla veriler modellenerek yararlanılacak bilgilere dönüştürülürler. K-Star algoritması veri madenciliği, obje tanımlama ve kontrol sistemleri gibi birçok alanda kullanılmakta olan örnek tabanlı bir yaklaşımdır. Bu çalışmada Makroekonomik değişkenlerin Bitcoin fiyatlarını etkileme seviyeleri, Makine Öğrenme yöntemlerinden Lazy Learning Öğrenmeye Dayalı K-Star Algoritması kullanılarak analiz edilmiştir. Çalışmanın veri seti, bağımlı ve bağımsız değişkenlerin 3 Ocak 2017 - 30 Ocak 2019 yılları arasındaki iş günü bazında 510 adet gözlem değerini içermektedir. Bu gözlemlerin 474 adedi (%93’ü) algoritmanın modellenmesi (eğitim) için, 36 adedi (%7’si) ise sınıflandırma (test) için kullanılmıştır. Modelin Bitcoin fiyatlarını gelecek dönem “yükseliş” mi yoksa “düşüş” mü göstereceğine ilişkin sınıflandırma başarısının %61,1 oranında olduğu, Bitcoin fiyatlarının “yükseliş” göstereceğine ilişkin doğru sınıflandırma başarısının %71,42, “düşüş” göstereceğine ilişkin doğru sınıflandırma başarısının ise %46,66 olduğu tespit edilmiştir. Sonuç olarak Makine Öğrenme Tekniğinin belli bir performans gösterdiği ancak Bitcoin fiyatlarının öngörülebilirliğinin henüz beklentinin altında olduğu ortaya çıkmıştır.
Referanslar
- Adetiloye, T., ve Awasthi, A. (2017). “Predicting Short-Term Congested Traffic Flow on Urban Motorway Networks”, In Handbook of Neural Computation, Academic Press, 145-165.
- Atik, M., Köse, Y., Yılmaz, B. ve Sağlam, F. (2015). “Kripto para: Bitcoin ve Döviz Kurları Üzerine Etkileri”, Bartın Üniversitesi İİBF Dergisi, 6(11), 247-261.
- Baek, C. ve Elbeck, M. (2015). “Bitcoin as An Investment or Speculative Vehicle? A First Look”, Applied Economics Letters 22, No. 1, 30–34.
- Basarır, C., ve Bayramoğlu, M. F. (2018). “Global Macroeconomic Determinants of the Domestic Commodity Derivatives”. In Global Approaches in Financial Economics, Banking and Finance, Springer, Cham, 331-349.
- Bayramoğlu, A. T. ve Başarır, Ç. (2019). “The Linkage Between Cryptocurrencies and Macro-Financial Parameters: A Data Mining Approach. In Blockchain Economics and Financial Market Innovation”, Springer, Cham 249-269.
- Birattari, M., Bontempi, G. ve Bersini, H. (1999). “Lazy Learning Meets The Recursive Least Squares Algorithm. in Advances in Neural Information”, Processing Systems, 375-381.
- Bontempi, G., Birattari, M. ve Bersini, H. (1999). “Lazy Learning For Local Modelling and Control Design”, International Journal of Control, 72(7-8), 643-658.
- Bozic, N., Guy, P. ve Stefano, S. (2016). “A Tutorial on Blockchain and Applications to Secure Network Control-Planes’’, 3rd Smart Cloud Networks and Systems (SCNS), 1-8.
- Briere, M., Oosterlinck, K. ve Szafarz, A. (2015). “Virtual Currency, Tangible Return: Portfolio Diversification with Bitcoin”, Journal of Asset Management, 16(6), 365-373.
- Ceyhan, K., Kurtulmaz, E., Sert, O. C. ve Özyer, T. (2018). “Bitcoin Movement Prediction With Text Mining”. 2018 26th Signal Processing and Communications Applications Conference (SIU), IEEE, 1-4.
- Cheah, E. T., ve Fry, J. (2015). “Speculative Bubbles in Bitcoin Markets? An Empirical Investigation into the Fundamental Value of Bitcoin”, Economics Letters, 32-36.
- Cheung, A., Eduardo R. ve Jen-Je S. (2015). "Crypto-Currency Bubbles: An Application of The Phillips–Shi–Yu (2013) Methodology On Mt. Gox Bitcoin Prices", Applied Economics, Cilt 47, Sayı 23, s. 2348-2358.
- Chu, J., Nadarajah, S. ve Chan, S. (2015). “Statistical Analysis of The Exchange Rate of Bitcoin. PLoS One”, 10(7), 1–27.
- Cleary, J. G., ve Trigg, L. E. (1995). “K*: An Instance-Based Learner Using An Entropic Distance Measure”, In Machine Learning Proceedings, 108-114.
- Coşkun, C. ve Baykal, A. (2011). “Veri Madenciliğinde Sınıflandırma Algoritmalarının Bir Örnek Üzerinde Karşılaştırılması”, Akademik Bilişim, 1-8.
- Crosby, M., Nachiappan, P., Sanjeev V. ve Vignesh K. (2016). “Blockchain Technology: Beyond Bitcoin,”, Applied Innovation Reiew, Sayı 2, 6-19.
- Ferdiansyah, F. (2019). “A Study of Bitcoin Stock Market Prediction: Methods”, Techniques and Tools, 1-5.
- Greaves, A. ve Au, B. (2015). “Using The Bitcoin Transaction Graph to Predict The Price of Bitcoin”, No Data, Computer Science, 1-8.
- Hormozi, H., Hormozi, E., ve Nohooji, H. R. (2012). “The Classification of The Applicable Machine Learning Methods in Robot Manipulators”. International Journal of Machine Learning and Computing, 2(5), 560–563.
- Investing, 2019a, https://tr.investing.com/crypto/currencies, Erişim tarihi: 18.12.2019.
- Investing, 2019b, https://tr.investing.com/crypto/Bitcoin/historical-data, Erişim tarihi: 10.12.2019.
- İçellioğlu, C. Ş., ve Öztürk, M. B. E. (2018). “Bitcoin ile Seçili Döviz Kurları Arasındaki İlişkinin Araştırılması: 2013-2017 Dönemi İçin Johansen Testi ve Granger Nedensellik Testi”, Maliye ve Finans Yazıları, 1(109), 51-70.
- Jang H. ve Lee J. (2018). “An Empirical Study On Modeling and Prediction of Bitcoin Prices with Bayesian Neural Networks Based on Blockchain”, Information IEEE Access, 6, 5427-5437.
- Joshi, A. P., Han, M., ve Wang, Y. (2018). “A Survey on Security And Privacy Issues of Blockchain Technology”, Mathematical Foundations of Computing, 1(2), 121-147.
- Kaplanov, N. M. (2012). “Nerdy Money: Bitcoin, The Private Digital Currency, and The Case Against Its Regulation”, Temple Law Review, 111-157.
- Karasu, S., Altan, A., Saraç, Z., ve Hacioğlu, R. (2018). “Prediction of Bitcoin Prices with Machine Learning Methods Using Time Series Data. 26”, Signal Processing and Communications Applications Conference (SIU) IEEE, 1-4.
- Kartal, C. ve Bayramoğlu M.F. (2019). “Blockchain Economics and Financial Market Innovation: Forecasting the Prices of Cryptocurrencies Using GM(1,1) Rolling Model”, Springer, 2019, 201-230.
- Khan, M. A. ve Salah, K. (2018). “Iot Security: Review, Blockchain Solutions, and Open Challenges”, Future Generation Computer Systems, 82, 1-32.
- Mallqui, D. C., ve Fernandes, R. A. (2019). “Predicting The Direction, Maximum, Minimum and Closing Prices Of Daily Bitcoin Exchange Rate Using Machine Learning Techniques”, Applied Soft Computing, 75, 596-606.
- Painuli, S., Elangovan, M. ve Sugumaran, V. (2014). “Tool Condition Monitoring Using K-Star Algorithm”, Expert Systems with Applications, 41(6), 2638-2643.
- Radityo, A., Munajat Q. ve Budi, I (2017). “Prediction of Bitcoin Exchange Rate to American Dollar Using Artificial Neural Network Methods”, Advanced Computer Science and Information Systems (ICACSIS), International Conference, 433–438.
- Swan, M. (2015). Blockchain: Blueprint for a New Economy. O'Reilly Media, Inc.
- Şahin, E. E. (2018). “Kripto Para Bitcoin: ARIMA ve Yapay Sinir Ağları ile Fiyat Tahmini”, Fiscaoeconomia, 2(2), 74-92.
- Tschorsch, F. ve Björn, S. (2016); “Bitcoin and Beyond: A Technical Survey on Decentralized Digital Currencies”, IEEE Communications Surveys & Tutorials’’, Cilt 18, sayı 3, 2084-2123.
- Underwood, S. (2016).” Blockchain Beyond Bitcoin”, Communications of The ACM, 59(11), 15-17.
- Usta, A. ve Doğantekin, S. (2017). Blokchain 101, MediaCat Kitapları. İstanbul.
- Viera, A. J. ve Garrett, J. M. (2005). “Understanding Interobserver Agreement: The Kappa Statistic”. Fam med, 37(5), 360-363.
- Vijayarani, S. ve Muthulakshmi, M. (2013). “Comparative Analysis of Bayes And Lazy Classification Algorithms”, International Journal of Advanced Research in Computer and Communication Engineering, 2(8), 3118–3124.
- Zheng, Z., Xie, S., Dai, H., Chen, X., ve Wang, H. (2017). “An Overview of Blockchain Technology: Architecture, Consensus, and Future Trends”, In Big Data (BigData Congress), IEEE Internationa