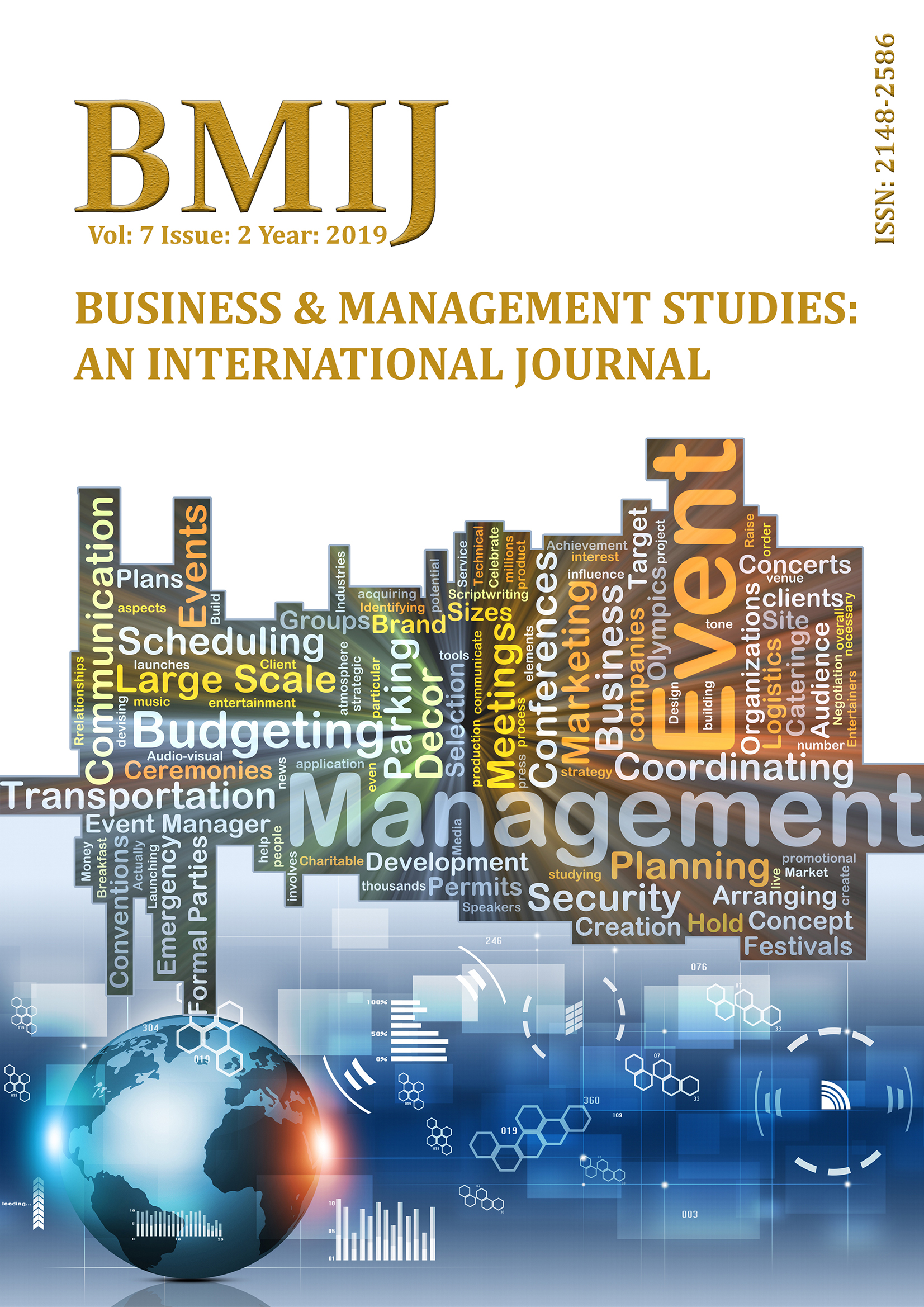
Yayınlanmış 26.06.2019
Anahtar Kelimeler
- K-means Clustering, Cluster Analysis, Initial Cluster Centers
- K-ortalamalar Kümeleme Yöntemi, Kümeleme Analizi, Başlangıç Merkez Noktası
Nasıl Atıf Yapılır
Nasıl Atıf Yapılır
Öz
K-ortalamalar kümeleme yöntemi, belirli bir veri kümesindeki birimleri önceden belirlenmiş sayıda kümeye ayıran en basit, denetimsiz öğrenme algoritmalarından biridir. Bu yöntem diğer iteratif yöntemler gibi başlangıç olarak seçilen ve küme merkezi olarak atanan değer veya değerlere bağlı kalarak bir kümeleme gerçekleştirir. K-ortalamalar yönteminde; ilk adımı rastlantısal olarak seçilen küme merkezleri yardımıyla, veri kümesindeki tüm birimlerin bu merkez noktalara olan uzaklıkları dikkate alınarak, birimlerin ait olduğu kümeler belirlenir. Bu rastlantısal olarak seçilen küme merkezleri farklı küme yapıları oluşturabilmektedir. Bu çalışma da başlangıç küme merkezi seçim sorunsalının varlığının daha detaylı anlaşılması adına, sorunsalın gözlemlendiği bir kurgu çalışma oluşturulmuştur. Kurgu çalışmada birimlerin iki ve üç kümeye ayrışmak istendiği durum için, veriler öncelikli olarak veri setinde yer alan tüm olası başlangıç merkez verilerle k-ortalamalar kümeleme yöntemi uygulanarak ayrıştırılmış ve farklı küme yapılarının farklı sıklıklarla elde edildiği gözlemlenmiştir. Ayrıca sorunsalın varlığını daha detaylı incelemek adına, veri setine yakın ve uzak konumlarda olacak şekilde veri setinde yer almayan yeni birimler oluşturabilmek için bir yöntem geliştirilmiştir. Daha sonra yöntemle elde edilen yeni birimler, başlangıç merkez veri olarak ele alınarak, veri seti kümelere ayrılmış ve daha önce elde edilmeyen yeni küme yapıları gözlemlenmiştir. Çalışmanın son kısmında ise başka bir kurgu çalışma ile veri seti içinden veya veri seti dışından seçilen başlangıç merkez birimlerle farklı sonuçlar elde edilebileceği gösterilmiştir.
Referanslar
- Akay, Ö. (2019). “Türkiye’de Halk Kütüphanesi Kullanımının Panel Veri Kümeleme Analizi İle İncelenmesi”, Uluslararası Toplum Araştırmalar Dergisi, 10(17), 1076-1099.
- Akçapınar, G., Altun, A., & Aşkar, P. (2016). “Çevrimiçi Öğrenme Ortamındaki Benzer Öğrenci Gruplarının Kümeleme Yöntemi İle Belirlenmesi”, Eğitim Teknolojisi Kuram ve Uygulama, 6(2), 46-64.
- Aydın, N. & Seven, A.N. (2015). “İl Nüfus Ve Vatandaşlık Müdürlüklerinin İş Yoğunluğuna Göre Hibrid Kümeleme İle Sınıflandırılması”. Yönetim ve Ekonomik Araştırmalar Dergisi, 13(2), 181-201.
- Atalay, A., & Tortum, A. (2010). “Türkiye'deki İllerin 1997-2006 Yılları Arası Trafik Kazalarına Göre Kümeleme Analizi”. Pamukkale University Journal of Engineering Sciences, 16(3), 335-345.
- Bülbül, Ş., & Camkıran, C. (2018). “Bankaların Klasik ve Bulanık Yaklaşımlarla Sınıflandırılması”. Trakya University Journal of Social Science, 20(2), 367-385.
- Çalışkan, S. K., & Soğukpınar, İ. (2008). “KxKNN: K-Means ve K En Yakın Komşu Yöntemleri İle Ağlarda Nüfuz Tespiti”. EMO Yayınları, 120-24.
- Çınaroğlu, S., & Bulut, H. (2018). “K-Ortalamalar ve Parçacık Sürü Optimizasyonu Tabanlı Kümeleme Algoritmaları İçin Yeni İlklendirme Yaklaşımları”. Gazi Üniversitesi Mühendislik-Mimarlık Fakültesi Dergisi, 33(2), 413-424.
- Duran, B. S. and P. L. Odel (1974). “Cluster Analysis (Lecture Notes in Economics and Mathematical Systems”, Econometrics; Managing Editors: M. Beckmann and H. P. Kunzf. Springer Verlag: NewYork.
- Durucasu, H., Aşan, Z., & Er, F. (2006). “Öğrencilerin Yaz Okulu Hakkındaki Görüşleri İçin Kümeleme Analizi”. Anadolu Üniversitesi Bilim ve Teknoloji Dergisi, 7(1), 97-101.
- Fırat, M., Dikbaş, F., Koç, A. C., & Güngör, M. (2012). “K-Ortalamalar Yöntemi İle Yıllık Yağışların Sınıflandırılması Ve Homojen Bölgelerin Belirlenmesi”. İMO Teknik Dergi, 383, 6037-6050.
- Forgy E.W. (1965). “Cluster Analysis of Multivariate Data: Efficiency vs. Interpretability of Classifications, Biometrics, 21 (3), 768-769.
- Fraley, C., & Raftery, A. E. (1998). “How Many Clusters? Which Clustering Method? Answers via Model-based Cluster Analysis”. The Computer journal, 41(8), 578-588.
- Khan, S. S., & Ahmad, A. (2013). “Cluster Center İnitialization Algorithm For K-Modes Clustering”. Expert Systems with Applications, 40(18), 7444-7456.
- Hajizadeh, E., Ardakani, H. D., ve Shahrabi, J. (2010). “Application of Data Mining Techniques ın Stock Markets: A Survey”. Journal of Economics and International Finance, 2(7), 109.
- Han, J., and Kamber, M., (2006), Data Mining Concepts and Techniques, Morgan Kauffmann Publishers Inc.
- Işık, M., & Çamurcu, A. Y. (2007). K-means, K-medoids ve bulanık C-means algoritmalarının uygulamalı olarak performanslarının tespiti.
- Jain, A. K., & Dubes, R. C. (1988). Algorithms for clustering data. Vol:6. Englewood Cliffs: Prentice hall
- Karypis, M. S. G., Kumar, V., & Steinbach, M. (2000, August). “A comparison of document clustering techniques”. In TextMining Workshop at KDD2000 (2000).
- Mac Queen, J.B., (1967). “Some Methods for Classification and Analysis of Multivariate Observations”. In: Proceedings of the Symposium on Mathematics and Probability, 5th, Berkely.
- Meila, M., & Heckerman, D. (2013). “An experimental comparison of several clustering and initialization methods”. arXiv preprint arXiv:1301.7401.
- Mercer D. P., (2003). “Clustering Large Datasets”, http://www.stats.ox.ac.uk/∼ mercer/documents/Transfer.pdf (date accessed: 03.21.2011).
- Na, S., Xumin, L., & Yong, G. (2010, April). “Research on k-means clustering algorithm: An improved k-means clustering algorithm”. In 2010 Third International Symposium on intelligent information technology and security informatics (pp. 63-67). IEEE.
- Özdemir, A., & Orçanlı, K. (2012). “İki Aşamalı Kümeleme Algoritması İle Pazar Bölümlemesi, Müşteri Profillerinin Belirlenmesi ve Niş Pazarların Tespiti”. Uşak Üniversitesi Sosyal Bilimler Dergisi, (11).
- Higgs, R. E., Bemis, K. G., Watson, I. A., & Wikel, J. H. (1997). “Experimental designs for selecting molecules from large chemical databases”. Journal of Chemical Information and Computer Sciences, 37(5), 861-870.
- Selvi, H. Z., Çağlar, B. (2016). “Using K-Means and K-Medoids Methods for Multivariate Mapping”, International Journal of Applied Mathematics, Electronics and Computers, 4, 342-345.
- Steinley, D., & Brusco, M. J. (2007). “Initializing K-means Batch Clustering: A Critical Evaluation of Several Techniques”. Journal of Classification, 24(1), 99-121.
- Tatlıdil, H. (1992). “Uygulamalı Çok Değişkenli İstatistiksel Analiz”, H.Ü. Fen Fakültesi İstatistik Bölümü, Ankara.
- Witten I. H., Frank E., (1999), “Data Mining: Practical machine learning tools with Java implementations”, San Francisco, Morgan Kaufmann.
- Yalçın, S., & Ayyıldız, E (2018). “Analysis of Airports Using Clustering Methods: Case Study In Turkey”. Journal of Management Marketing and Logistics, 5(3), 194-205.
- Yaraş, E. (2005). “Tüketicilerin Pazarlama Karması Kararları Ve Marka Değeri Algılamaların Göre Kümeler Halinde İncelenmesi”. Atatürk Üniversitesi İktisadi ve İdari Bilimler Dergisi, 19(2), 349-372.
- Yedla, M., Pathakota, S. R., & Srinivasa, T. M. (2010). “Enhancing K-Means Clustering Algorithm with Improved Initial Center”. International Journal of computer science and information technologies, 1(2), 121-125.
- Zırhlıoğlu, G ve Karaca, S., (2006). “Genç Bayanlar Dünya Voleybol Şampiyonasına Katılan Sporcuların Kümeleme Analizi İle İncelenmesi”. Hacettepe J. Of Sport Sciences, 17(1): 20-25.